This publication is also available online in HTML in print-ready format at ic.gc.ca/smeresearch.
Aussi offert en français sous le titre Qu'est-ce qui stimule la croissance des entreprises? Analyse de régression quantile portant sur les petites et moyennes entreprises canadiennes – 2020.
To obtain a copy of this publication or an alternate format (Braille, large print, etc.), please fill out the Publication Request form or contact:
Web Services Centre
Innovation, Science and Economic Development Canada
C.D. Howe Building
235 Queen Street
Ottawa, ON K1A 0H5
Canada
Telephone (toll-free in Canada): 1-800-328-6189
Telephone (Ottawa): 613-954-5031
TTY (for hearing-impaired): 1-866-694-8389
Business hours: 8:30 a.m. to 5:00 p.m. (Eastern Time)
Email: ised@canada.ca
Permission to Reproduce
Except as otherwise specifically noted, the information in this publication may be reproduced, in part or in whole and by any means, without charge or further permission from the Department of Industry, provided that due diligence is exercised in ensuring the accuracy of the information reproduced; that the Department of Industry is identified as the source institution; and that the reproduction is not represented as an official version of the information reproduced, or as having been made in affiliation with, or with the endorsement of, the Department of Industry. For permission to reproduce the information in this publication for commercial purposes, please fill out the Application for Crown Copyright Clearance or contact the Web Services Centre (see contact information above).
Note: In this publication, the masculine form refers to both women and men.
© Her Majesty the Queen in Right of Canada, as represented by the Minister of Industry, 2020
Charles Bérubé and Patrice Rivard
Innovation, Science and Economic Development Canada
Small Business Branch
Research and Analysis Directorate
Table of contents
Summary
Firm growth is a key driver of economic growth and development by its fundamental association with job creation and its capacity to enhance aggregate productivity growth and living standards. Understanding the underlying process of firm growth has important policy implications as it can help policy-makers design more targeted policies to remove barriers and promote firm growth. This leads to the question: What drives firm growth? This report attempts to answer that question by studying determinants of firm employment growth over the period 20112013. This research uses Statistics Canada's Survey on Financing and Growth of Small and Medium Enterprises, 2011 linked to administrative data. The survey provides information on a comprehensive set of determinants, including firm characteristics (e.g., age, size, exports, innovation) and owner characteristics (e.g., age, education), to examine possible determinants of firm growth. Overall, estimations and results obtained by quantile regression techniques show asymmetries between rapidly shrinking firms and rapidly growing firms (high-growth firms).
Our empirical findings suggest that investments in research and development (R&D) and innovation — development or introduction of a new or significantly improved good or service, or a production process or method — have a positive and significant impact on rapidly growing firms. Investments in machinery and equipment (M&E), spending on training employees and owners' level of education have a positive impact on rapidly shrinking firms and high-growth firms, with the magnitude of the effects greater for the former. This means that those drivers could help firms that struggle a lot. Excessive debt, in comparison with assets (debt ratio), could hamper firm growth, specifically for rapidly shrinking firms compared with rapidly growing firms. Greater liquidity is another driver of firm growth, having a positive and significant impact on rapidly shrinking firms. Finally, owner motivation to grow in the future is also an important driver of firm growth for both rapidly shrinking firms and high-growth firms. We found that the magnitude of the effects is greater for the former. This suggests that owner characteristics, such as entrepreneur motivation, is a key variable for firm growth.
1. Introduction
Innovation, Science and Economic Development Canada's (ISED) mission "is to foster a growing, competitive, knowledge-based Canadian economy." The keyword in this mission statement is certainly "growing" and the underlying principle is "sustainable." In "An Essay on the Principle of Population," Malthus (1798) observed that economic growth was not sustainable as an increase in a nation's production, while temporarily improving the social well-being of the population, led to population growth instead of maintaining a higher standard of living.Footnote 1
Nowadays, modern economies have a propensity to take advantage of economic growth to improve citizens' standard of living, as expressed in ISED's mandate "to help make Canadian industry more productive and competitive in the global economy, thus improving the economic and social well-being of Canadians."
Stable and sustainable economic growth and development is thus the main policy objective of most advanced economies. Of course, there are other important policy objectives (e.g., stable prices, full employment, fair distribution of income, etc.) and not all economists agree about the order of priority for achieving these objectives (or, for that matter, the instruments that should be used to achieve a given objective). Everyone agrees, however, that economic policies are a deliberate attempt to generate increases in economic welfare. Business growth, as well as job creation, are certainly part of most governments' fundamental concerns and policies.Footnote 2
As the main rationale for growth economic research is to figure out the mechanism underlying economic growth, understanding those factors that have an impact on firm growth is, therefore, essential to provide efficient policies. Thus, our research question is at the centre of policy-makers' attention: What are the characteristics that enable a firm to make extraordinary contributions to growth and job creation? While a recently increasing body of studies has examined which types of firms create more jobs, little is known about the firm growth process and the determinants of growth in the Canadian context.
2. Literature review and policy perspective
Empirical literature on firm growth is vast and involves different research areas, such as entrepreneurship, management and firm dynamics in general. Specific factors shaping firm growth have been studied,Footnote 3 with recent evidence highlighting the importance of business start-ups and young firms in job creation (Calvino et al., 2016; Haltiwanger et al., 2016). However, Decker et al. (2016) showed that the contribution of start-ups and young firms declined in the United States after 2000 and Acs et al. (2008) observed that the average high impact firm is around 25 years old when it makes a significant contribution to the economy. Lawless (2014) observed that "younger firms are consistently more dynamic than older firms," albeit there is "a strong inverse relationship between employment growth and firm size for young firms." However, this inverse relationship seems to decline significantly for older firms. According to Lawless (2014), "this provides some support for the Gibrat's law prediction that size and growth are independent, but only once the firm has moved beyond the start-up stage." We will come back with a more detailed review of Gibrat's law and its implication in Section 4.1.
Related work on assessing the dynamism of firms has also brought to light that a small number of firms, often labelled "high-growth firms" (HGFs),Footnote 4 represent a small fraction of the overall firm population while being disproportionately responsible for net job creation (Birch, 1979). Moreover, Coad et al. (2011, 2014) also presented evidence that HGFs create job opportunities for those who have difficulty finding employment (e.g., youth, immigrants, dropouts), thus increasing the social impact of HGFs through many positive externalities. HGFs are not the specific target of this paper, but they are part of the study and certainly worth a little digression.
At first, HGFs look like ideal targets for policy-makers. Their importance in terms of job creation likely triggered a cascade of studiesFootnote 5 seeking to identify conditions that would spark the creation and development of HGFs. Yet, apart from established stylized facts that HGFs tend to be younger, smallerFootnote 6 and omnipresent in all industries (Schreyer, 2000; Henrekson and Johansson, 2010), there is little evidence that would allow anyone to predict which firms will or could become HGFs. In fact, most empirical evidence tends to show that there is no way to predict this state, almost as if high growth has an inherent random success element (i.e., a coincidence of success factors with no priors of method), giving some assertion to Barney's (1997) random success argument. From a policy perspective, particularly in the context of how best to develop the Canadian "high-growth agenda," these results lend credence to the long-term inefficiency of potential targeted government programs aiming to influence the number of HGFs per say. However, there is a big caveat to reaching this conclusion concerning the concept and definitions, as well as the measures, of HGFs.
First, firm growth may be measured in terms of employment, sales, productivity and profit (or, in some rare cases, value added). Employment is probably the most adopted measure in studies as firm growth creates job opportunities and is often the focus of policy-makers. This is the measure we selected for this study. Second, HGFs can be identified using different definitions or thresholds:
- Organisation for Economic Co-operation and Development (OECD) definition:Footnote 7 Firms with 10 or more employees that have average annualized growth greater than 20 percent per year over a 3-year period, as measured by employment levels or employee turnover.
- Bureau of Labor Statistics (BLS) definition:Footnote 8 OECD definition for firms with 10 or more employees plus any firm with fewer than 10 employees that grows by eight or more employees over a 3-year period.
- Other definitions:Footnote 9 Researchers can adopt whatever threshold they want. Most of the time, HGFs are defined as the top quantile (top 10 percent and sometimes 5 percent or 1 percent) of firm growth distribution over a specific period.
Using the OECD or BLS definitions of HGFs,Footnote 10 one can instantly see that it is almost futile to try to develop policies that would attempt to influence the absolute number of HGFs as firms achieving high growth change from period to period (i.e., HGF status is not permanent by definition). The other definitions are no more useful in that sense because they are relative growth measures where the top 10 percent will always be 10 percent of the distribution! To raise the absolute number of HGFs using a relative definition would imply raising the population of firms. Moreover, the top 10 percent of firms displaying growth could display negative growth in theory.
Nevertheless, persistence of firm growth is particularly interesting for HGFs as policy-makers want to know if a firm's fast growth is likely to repeat itself, which brings us to the persistence question about HGFs and firm growth dynamics in general. The literature (using relative measures) shows some evidence that persistent HGFs do exist, although they are often characterized only in terms of size and age, with mixed results. Using a 1 percent threshold, Daunfeldt and Halvarsson (2015) showed that "HGFs are essentially 'one-hit wonders,' and it is thus doubtful whether policy-makers can improve economic outcomes by targeting them." A more absolute definition of HGFs, such as the OECD or BLS definitions, would likely demonstrate the same outcome, i.e., HGFs do not replicate their high-growth pattern over time. The results might differ with a less restrained measure of HGFs. A more advanced discussion on the subject of persistence is presented in Section 4.7.
3. Data
Specific factors shaping firm growth can roughly be grouped into three categories: characteristics related to the firm and those related to the owner and the employees. Characteristics related to the firm (e.g., firm age and size, industry sector, access to financial capital, export status, etc.) are collected by most national statistical agencies. Most of the time, characteristics related to the owner (e.g., age, education, gender, immigrant status, etc.) elude large analytical statistical databases and many studies rely upon specific entrepreneurship surveys. Unfortunately, those surveys are conducted to answer specific questions and often do not contain enough information on firm characteristics. Characteristics related to employees (e.g., education, experience, etc.) are even scarcer or non-existent in large statistical databases.
To obtain as much information as possible on firm and owner characteristics, we asked Statistics Canada to link the Survey on Financing and Growth of Small and Medium Enterprises, 2011 to the General Index of Financial Information (GIFI) and Payroll Account Deductions and Remittances (PD7) from 2008 to 2014. The Survey on Financing and Growth of Small and Medium Enterprises, 2011 was designed to determine the types of financing that small and medium-sized enterprises (SMEs) are using, and to collect information on recent attempts by those SMEs to obtain new financing. In addition, the survey gathers information on owner characteristics, engagement in international business activities and innovation. The linkage between the cross-sectional survey and the financial statements included in the General Index of Financial Information and Payroll Account Deductions and Remittances data allows us to assess firm performance over time, in particular firm growth.
For this report, we studied firm growth over the period 20112013.Footnote 11 The initial sample contained 9,977 observations. After cleaning and removing outliers and observations due to missing variables, 7,912 observations remained. A total of 958 observations exited the market, i.e., businesses that had zero employees or did not report any information on employment in 2013. Thus, we ended up with a sample of 6,954 observations for our analytical framework.
4. Analytical framework
4.1 Firm growth rate distribution
Firm growth rate distribution has been extensively studied over the years. Coad (2007a) noted that, historically, the study of firm growth rate distribution started with the work of Ashton (1926) and later Little (1962). In particular, both authors found that growth rate distributions are fat-tailed. Gibrat (1931) was one of the first to propose a theoretical model to explain firm growth. He formulated the well-known Law of Proportionate Effect, also known as Gibrat's law. This law stipulates that firm growth is a random process. Gibrat's law also implies that firm growth rates are independent of firm size at the beginning of the period (Lotti et al., 2003).
We modelled Gibrat's law using Sutton's (1997) and Coad's (2007a) presentation. Let denote the size of a firm at and , the proportionate rate of growth between and . From Gibrat's law,
where can be interpreted as an independent random shock. We can rewrite the previous equation as
,
which leads to
,
with denoting the initial firm's size. Then, by taking the logarithms, we have
,
where we assume that for sufficiently small. As a consequence,Footnote 12
.
Therefore, firm growth is a result of the sum of small independent shocks. By assuming that the shocks are independent and identically distributed, the Central Limit Theorem implies that approximately follows a normal or Gaussian distribution (i.e., the distribution of is log-normal). This holds for any distribution of .
A consequence of Gibrat's law is that smaller firms do not grow faster than larger firms. Gibrat's law has been tested empirically by many researchers. Older studies, such as Hart and Prais (1956), Simon and Bonini (1958) and Hymer and Pashigian (1962), attempted to confirm Gibrat's law. However, a large number of recent studies have shown that Gibrat's law does not fit empirical results (i.e., that firm size is statistically related to firm growthFootnote 13).
Stanley et al. (1996), followed by Bottazzi et al. (2001), empirically investigated the distribution of firm growth. They showed that the distribution is non-Gaussian, corresponding instead to a Laplace distribution.Footnote 14 Thus, a firm's growth rate distribution is tent shaped with fat tails, which does not correspond to a Gaussian distribution. Many other empirical studies from various countries, using multiple datasets, have reached similar conclusions (Reichstein and Dahl, 2004; Bottazzi and Secchi, 2005a, b; Reichstein and Jensen, 2005; Bottazzi et al., 2011; Erlingsson et al., 2012). The tent-shaped form of the distribution is robust to the industry sector, which allows us to conclude that the Laplace distribution of firm growth rate is not an aggregation effect (Bottazzi and Secchi, 2005a, b; Bottazzi et al., 2011).
In Canada, firm growth rate distribution has been explored by Dixon and Rollin (2014). They found that Canadian firm growth rate distribution also follows a Laplace distribution. The authors considered employment growth rate using Statistics Canada's Longitudinal Employment Analysis Program database over the 20002009 period. They showed, in particular, that the employment growth rate distribution has more density in the centre and in the tails than a Gaussian distribution. Moreover, the employment growth rate distribution shows the same shape among all industry sectors.
Given the fact that Gibrat's law does not fit empirical data very well, Bottazzi and Secchi (2003a) suggested another theoretical model by modifying some of the assumptions involved in the model proposed by Gibrat. An important assumption behind Gibrat's law implies that the growth process is independent among firms. In other words, it is assumed that there is no competition among firms (Bottazzi and Secchi, 2005a). The authors introduced competition into the model by assuming that there are a finite number of opportunities available to firms. Thus, competitive behaviour follows from the availability of limited resources that firms try to seize (Bottazzi and Secchi, 2005b). Within this context, firm growth is linked to the number of opportunities that a firm is able to capture. For example, as suggested by Bottazzi and Secchi (2005a), opportunities could take various forms as new technology exploitation, demand shock adjustments or managerial reorganization effects.
In addition to the assumption of a finite number of opportunities among firms, it is also assumed that a firm's probability of obtaining new opportunities is related to the number of opportunities already obtained. According to Bottazzi and Secchi (2005b), this introduces "dynamic increasing returns in the growth process of firms." Economies of scale or knowledge accumulation are examples of mechanisms leading to dynamic increasing returns in a firm's growth process (Bottazzi and Secchi, 2003a, b). This form of competition, introduced in the BottazziSecchi growth dynamic model, leads to a form of firms' attraction effect to opportunities, which generates a fat-tailed distribution (Bottazzi and Secchi, 2005b).
Using these assumptions and a Pólya urn scheme,Footnote 15 Bottazzi and Secchi (2003a) have shown that a firm's growth distribution is asymptotically a Laplace distribution. The authors also used simulations to reproduce firms' growth rate distribution and observed that the distribution is tent shaped.
4.2 Quantile regression
The previous section has demonstrated how far a firm's growth distribution is from a Gaussian distribution. In particular, the distribution shows heavy tails, indicating that firms with extreme growth rates exist more often than would be the case based upon a Gaussian distribution. In other words, a firm's growth rate distribution displays the presence of many outliers. The non-Gaussian nature (i.e., non-normality) of firm growth rates suggests that ordinary least squares (OLS) regression may not be well suited to investigate growth determinants within this context. OLS regressions estimate the average effect on the dependent variable due to a change in the independent variables, thus putting the focus on the "average firm" (Coad and Rao, 2008). This implies that the relationship between the explanatory variables and the dependent variable (i.e., firm growth) is fixed across the distribution. In other words, the estimated coefficients of the model do not change with the values of the variables and will not reflect the tails of the distribution (i.e., firm growth behaviour).
Because the impact of explanatory variables may vary at different points of the growth distribution (Mathew, 2017), quantile regressionFootnote 16 provides a better tool for studying firm growth than OLS regressions. Quantile regression takes into account the effects of the explanatory variables (i.e., the estimated coefficients of the model) at different points of the dependent variable distribution and not solely in terms of the conditional mean. This implies that we can specifically assess how determinants could have a different impact on firm growth based upon where their growth is situated in the distribution. It is implicitly assumed, however, that an observation (i.e., firm) stays in the same (growth) quantile of the distribution (Cameron and Trivedi, 2010). In any case, quantile regression provides a complete picture by exploring all parts of the distribution of the dependent variable (Falk, 2012). Another advantage of the model is its robustness to the presence of outliers and to fat-tailed distributions (Coad and Rao, 2008; Reichstein et al., 2010). The popularity of quantile regressions has increased over the past years and many researchers have used this econometric model to study firm growth distribution.Footnote 17
In the following, we adopt the notation of Cameron and Trivedi (2005). For any choice of a quantile , such that , the quantile regression estimators are calculated by minimizing the following function:
.
For different choices of , we obtain different values of the estimators . Counterintuitively, quantile regressions are not separately calculated on quantile subsamples of the initial distribution. Quantile regressions use all observations that are weighted through the function that is minimized. Interpretation of the estimated coefficient in a quantile regression is similar to that in an OLS regression. It corresponds to the marginal effect, i.e., the partial derivative of the conditional quantile of the dependent variable with respect to a particular explanatory variable. In other words, it is the marginal change in the outcome at the th quantile due to a small change in the chosen explanatory variable (Coad and Rao, 2008).
4.3 Inverse probability weighting approach
The main objective of this report is to study the determinants of firm growth over the period 20112013. We use firm size as a measure of growth and growth is calculated as the logarithmic difference in size between 2011 and 2013,
.
Growth was not calculated for firms with no employees or when the number of employees was not available. For example, this was the case for firms that did not report information on employment in 2013. As a consequence, firm growth was calculated only for surviving firms. As mentioned in Section 3, from the 7,912 businesses in the sample in 2011, 958 businesses exited the market in 2013, representing 12 percent of the total number of observations in the sample.
The sample of firms was not randomly selected as firms were chosen that had at least one employee. Potentially, this could create a deficiency in the results by introducing selection bias (Haltiwanger et al., 2016). This could happen when the missing observations are related to the dependent variable (Greene, 2012). As a result, estimated coefficients could be biased and inconsistent (Stucki, 2013). The following shows how we can calculate consistent estimators despite the potential bias introduced through the selection process.
Following Fitzgerald et al. (1998), let and be the dependent variable and the covariates respectively. Suppose that we want to estimate the next equation:
. (1)
Let be a dummy variable related to the selection of the observations and let if the observation is selected and 0 otherwise. A firm is selected if it has at least one employee. On the other hand, a firm is not selected if it has zero employees or if information on employment is missing.Footnote 18 Thus, is observed when . Let
.(2)
Fitzgerald et al. (1998) introduced the notion of selection on unobservables and selection on observables relative to conditions on some terms in equations (1) and (2).
Selection on unobservables occurs when, conditional on :
1. is independent of
2. is not independent of .
The first assumption means that the variable is not related to through unobservables (i.e., is not endogenous to the outcome variable ). The second assumption suggests that the selection process is correlated with the outcome variable or the selection process is correlated with unobservables after conditioning on covariates (Wooldridge, 2002). As formulated by Wooldridge (2002), the term "unobservable" means that selection is correlated with part of the dependent variable that is not explained by the covariate . Selection on unobservables is related to the Heckman (1979)Footnote 19 correction method, which is frequently used in the context of selection bias correction. The Heckman modelFootnote 20 necessitates, among other things, finding at least one variable () that has an impact on the probability of selection (), but does not affect the outcome variable (i.e., ). Not having this exclusion restriction (Cameron and Trivedi, 2010) could lead to collinearity problems in the estimation of the two-step Heckman procedure, especially in small samples (Puhani, 2000; Cameron and Trivedi, 2005) because if affects outcome , it would mean that is correlated to , thus the collinearity.
Selection on observables occurs when, conditional on :
- is not independent of
- 2. is independent of .
The first assumption means that the variable is endogenous to the outcome variable . The second assumption can be interpreted as should not be related to unobservables that affect . These assumptions could be interpreted as follows:
.
This is called the ignorability assumption. Thus, conditional on , selection (or attrition) can be treated as ignorable non-response (Contoyannis et al., 2004). Fitzgerald et al. (1998) pointed out the necessity to find a variable that is a good predictor of selection, but does not appear in the "structural" equationFootnote 21 related to in equation (1). Wooldridge (2002) also noted that has to be a good proxy of unobservables that affect (i.e., assumption 1) and, of course, also be able to predict selection. This is a major difference with the Heckman method, where the exclusion restriction variable is independent of the outcome variable . As mentioned by Jones et al. (2006), if the aforementioned conditions are satisfied, it is possible to obtain consistent estimators by using weights calculated from the inverse probability of an observation being selected (conditional on covariate ). This methodology is called the inverse probability weighting (IPW) estimator.Footnote 22
Essentially, the idea behind weighting places more importance on firms with a lower probability of being selected as they are under-represented in the sample. Therefore, using the inverse probability weighting estimator gives them more influence in the model (Stucki, 2013). Wooldridge (2002) showed that the IPW estimator is consistent with and asymptotic to the normal distribution (Jones et al., 2006). It is worth mentioning that the estimated standard errors without adjustment are, by definition, larger than the standard errors with adjustment.Footnote 23 Therefore, in this case, ignoring the adjustment is considered a "conservative inference" (Jones et al., 2006). This implies that significant estimates are obtained with smaller t-statistics than if we had used the correct standard errors. Standard errors are not adjusted in our study and the results can be considered as "conservative inference," meaning that significant estimates would have been even more significant using standard error adjustments. However, this could also mean that some significant variables could show up as insignificant.
We applied the IPW estimator using a two-step approach to control for selection bias. In the first step, we estimated the probability of a firm being selected, using a probit regression with covariates related to firm performance. In the second step, we used quantile regression to estimate the impact of variables on firm growth, using the weights calculated in the first step.Footnote 24
The IPW estimator has been adopted in many empirical works, such as Fitzgerald et al. (1998), Contoyannis et al. (2004) and Jones et al. (2006). In our context, where we study firms, the IPW estimator was used by Stucki (2013) and more recently by Haltiwanger et al. (2016). Some authors, such as Serrasqueiro et al. (2010), used the Heckman correction method to control for selection bias, and some, such as Navaretti et al. (2014), conducted analyses conditional on survival, although without controlling for sample selection.
4.4 Variables and descriptive statistics of the sample
In this study, all financial variables are measured in 2011 constant dollarsFootnote 25 to address the inflation factor. Table 1 presents the dependent variable and explanatory variables used in the quantile regressions. Explanatory variables fall into four categories: firm characteristics, barriers to growth, owner characteristics and macroeconomic factors. For the last category, the literature shows that firm growth could be sensitive to macroeconomic shocks (Reichstein and Jensen, 2005; Ipinnaiye et al., 2017). We control for that aspect by using unemployment rate and population growth. Also, some explanatory variables were averaged over the 20112012 period instead of the 20112013 period. This was done to avoid endogeneity introduced by potential simultaneity effects as the dependent variable (i.e., firm growth) is calculated over the 20112013 period.
Variable | Definition | |
---|---|---|
Dependent variable | Firm growth | Firm size (number of employees) growth between 2011 and 2013 measured as the difference, in logarithmic terms, in firm size in 2013 and firm size in 2011. |
Firm characteristics | Past firm growth | Yearly average of firm growth (difference in logarithmic terms) between 2009 and 2011. |
Firm age | Firm age in 2011, in logarithmic terms. | |
Firm size, Firm size squared | Number of employees in 2011, in logarithmic terms, and its squared term. | |
Sales growth | Growth (difference in log terms) of total sales of goods and services between 2011 and 2012. | |
Export | = 1 if the firm exported goods or services in 2011; = 0 otherwise. | |
Expected export | = 1 if the business intends to expand sales to new markets outside of Canada in the next 3 years; = 0 otherwise. | |
Research and development | = 1 if the value spent on R&D is nonzero; = 0 otherwise. | |
Machinery and equipment | = 1 if the value spent on new M&E is nonzero; = 0 otherwise. | |
Information and communication technology (ICT) | = 1 if the value spent on ICT is nonzero; = 0 otherwise. | |
Innovation (reference category: no innovation) | = 1 if the business developed or introduced, over the past 3 years, a new or significantly improved good or service, or a production process or methodFootnote 26; = 2 if the business developed or introduced a new organizational method in business practices, workplace organization or external relations, or new way of selling goods or services, but did not introduce improved goods or services, or a production process or method; = 0 otherwise. | |
Training | = 1 if the value spent on education and training for employees is nonzero; = 0 otherwise. | |
Capital, Capital growth | Level of capital (in logarithmic terms) in 2011 and growth (difference in log terms) over 20112012. | |
Wages, Wage growth | Level of total wages paid (in logarithmic terms) in 2011 and growth (difference in log terms) over 20112012. | |
Debt ratio | Current debt ratio calculated as total current liabilities divided by total assets and averaged over 20112012. | |
Liquidity | Liquidity (or cash flow) ratio calculated as total current assets divided by total assets and averaged over 20112012. | |
Return on assets (ROA) | Return on assets is calculated as the ratio of net income/loss after taxes divided by total assets and averaged over 20112012. | |
Industry (reference category: retail trade) | = 1 if the business is in one of the following sectors: agriculture, forestry, fishing and hunting; mining, quarrying, and oil and gas extraction; construction; manufacturing; wholesale trade; retail trade; transportation and warehousing; information and cultural industries; real estate and rental and leasing; professional, scientific and technical services; administrative and support, waste management and remediation services; health care and social assistance; arts, entertainment and recreation; accommodation and food services; other services; = 0 otherwise. | |
Provinces/territories (reference category: Ontario) | = 1 if the business is located in one of the following provinces, group of provinces or territories: Atlantic (New Brunswick, Nova Scotia, Prince Edward Island, Newfoundland and Labrador), Quebec, Ontario, Manitoba, Saskatchewan, Alberta, British Columbia, Territories (Yukon, Northwest Territories, Nunavut); = 0 otherwise. | |
Barriers to growth | Growth obstacles | Dummy variable for each of the following external obstacles (= 1 if the obstacle was a serious problem for the growth of the company; = 0 otherwise): shortage of labour, fluctuations in demand for products or services, obtaining financing, government regulations, rising cost of inputs, increasing competition, other; and for each of the following internal obstacles: managing debt level, maintaining sufficient cash flow, lack of monitoring business operations to make improvements, lack of knowledge about competitors or market trends, devoting too much time to day-to-day operations, recruiting and retaining employees, other. |
Owner characteristics | Age | Age of majority owner or general manager owning or managing a business, in logarithmic terms. |
Education | = 1 if the highest level of education attained by the majority owner or general manager is a bachelor's degree, master's degree or above; = 0 otherwise. | |
Motivation | = 1 if the expected average yearly growth in sales or total revenues over the next 3 years (20122014) is higher than 10 percent per year; = 0 otherwise. | |
Indigenous Peoples | = 1 if majority ownership of the business is held by an Indigenous person; = 0 otherwise. | |
Visible minority | = 1 if majority ownership of the business is held by a person from a visible minority group; = 0 otherwise. | |
Immigrant status | = 1 if the majority owner or general manager was born outside of Canada; = 0 otherwise. | |
Majority-female ownership | = 1 if the percentage of the business owned by a woman is more than 50 percent; = 0 otherwise. | |
Macroeconomic factors | Unemployment rate growth | Difference in unemployment rate between 2011 and 2012 by census metropolitan area (CMA). |
Population growth | Growth rate (difference in log terms) of population between 2011 and 2012 by CMA. |
Variable | Mean value* (standard deviation) |
---|---|
Past firm growth | 0.03 (0.21) |
Firm age | 24.06 (19.42) |
Firm size | 22.80 (42.29) |
Sales growth | −0.45 (2.39) |
Export (%) | 18.28 |
Expected export (%) | 18.88 |
Research and development (%) | 13.50 |
Machinery and equipment (%) | 46.61 |
Information and communication technology (%) | 46.22 |
No innovation (%) | 55.64 |
Innovation (products, processes or other) (%) | 34.80 |
Innovation (only organizational methods or new way of selling goods or services) (%) | 9.56 |
Training (%) | 45.59 |
Capital per employee ($) | 132,538 (455,164.5) |
Wages ($) | 44,516 (51,175.14) |
Debt ratio | 0.49 (0.80) |
Liquidity | 0.59 (0.29) |
Return on assets | 0.09 (0.50) |
Owner age | 52.08 (10.49) |
Education (%) | 35.35 |
Motivation (%) | 74.55 |
Indigenous Peoples (%) | 1.67 |
Visible minority (%) | 5.84 |
Immigrant status (%) | 15.01 |
Majority-female ownership (%) | 10.15 |
Unemployment rate growth (difference) | −0.26 (0.38) |
Population growth | 0.01 (0.01) |
Number of observations | 6,954 |
* For dummy variables, the proportion is reported instead of the mean.
Sources: Statistics Canada, Survey on Financing and Growth of Small and Medium Enterprises, 2011; General Index of Financial Information 20082014; and authors' calculations.
Industry | Percentage |
---|---|
Agriculture, forestry, fishing and hunting | 6.60 |
Mining, quarrying, and oil and gas extraction | 1.80 |
Construction | 9.48 |
Manufacturing | 14.29 |
Wholesale trade | 16.88 |
Retail trade | 7.82 |
Transportation and warehousing | 11.07 |
Information and cultural industries | 0.83 |
Real estate and rental and leasing | 1.37 |
Professional, scientific and technical services | 13.73 |
Administrative and support, waste management and remediation services | 3.22 |
Health care and social assistance | 2.63 |
Arts, entertainment and recreation | 1.02 |
Accommodation and food services | 2.83 |
Other services | 6.41 |
Total | 100 |
Sources: Statistics Canada, Survey on Financing and Growth of Small and Medium Enterprises, 2011; General Index of Financial Information 20082014; and authors' calculations.
Region | Percentage |
---|---|
Atlantic | 10.28 |
Quebec | 22.56 |
Ontario | 33.43 |
Manitoba | 5.09 |
Saskatchewan | 5.65 |
Alberta | 11.10 |
British Columbia and Territories | 11.88 |
Total | 100 |
Obstacle | Percentage | |
---|---|---|
External obstacles | Rising cost of inputs | 64.37 |
Fluctuations in demand for products or services | 52.56 | |
Increasing competition | 47.73 | |
Shortage of labour | 35.54 | |
Government regulations | 34.99 | |
Obtaining financing | 15.85 | |
Other external obstacles | 22.65 | |
Internal obstacles | Recruiting and retaining employees | 43.67 |
Devoting too much time to day-to-day operations | 39.63 | |
Maintaining sufficient cash flow | 38.16 | |
Managing debt level | 17.73 | |
Lack of monitoring business operations to make improvements | 17.34 | |
Lack of knowledge about competitors or market trends | 13.33 | |
Other internal obstacles | 9.59 |
Sources: Statistics Canada, Survey on Financing and Growth of Small and Medium Enterprises, 2011; General Index of Financial Information 20082014; and authors' calculations.
4.5 Firm growth distribution
We analyzed firm size growth over the period 20112013 and found that 30 percent of businesses in the sample did not grow at all. Investigation of firm size growth distributionFootnote 27 confirms that it is non-Gaussian (see Figure 1). The distribution is tent shaped and has heavy tailsFootnote 28 compared with the Gaussian distribution. This result is similar to what Coad (2007b), Coad and Rao (2008), Reichstein et al. (2010), Capasso et al. (2013) and Dixon and Rollin (2014) found in their studies of firm size growth distribution.
Figure 1: Firm size growth distribution
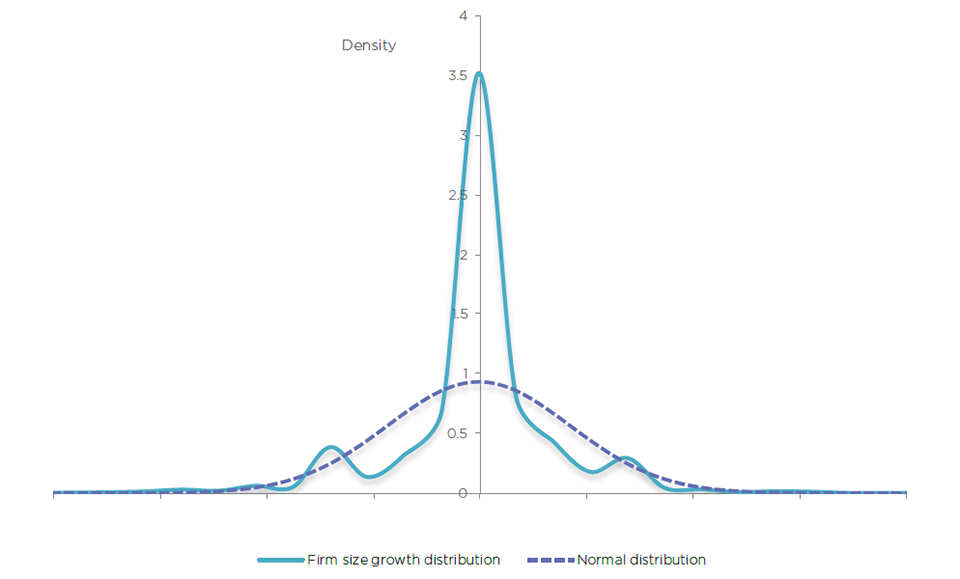
Sources: Statistics Canada, Survey on Financing and Growth of Small and Medium Enterprises, 2011; General Index of Financial Information 2008–2014; and authors' calculations.
We also performed another robustness checkFootnote 29 to determine to what extent the firm size growth distribution differs from a Gaussian distribution. Figure 2 displays a distributional diagnostic plot test that maps the quantiles of firm growth against the quantiles of a normal distribution. The quantiles of the normal distribution are represented by the straight black line. In particular, at the extremities we see that the distribution is far from Gaussian as the growth distribution tails are thicker.
Figure 2: Quantiles of the firm size growth distribution and the normal distribution

Sources: Statistics Canada, Survey on Financing and Growth of Small and Medium Enterprises, 2011; General Index of Financial Information 2008–2014; and authors' calculations.
4.6 Comparison of rapidly shrinking firms, non-growing firms and high-growth firms
The main purpose of this paper is to explore the possible drivers of SME growth. To do this, we have proposed a method involving quantile regressions, which allowed us to analyze the impact of various factors on firm growth (given their position on the growth distribution). More precisely, we were interested in firms that are located at the bottom (or left-hand side), middleFootnote 30 and top (or right-hand side) of the firm growth distribution.
These three groups correspond to rapidly shrinking firms (negative growth and located at the bottom of the distribution), non-growing firms (located in the middle of the distribution) and high-growth firms (positive growth and located at the top of the distribution). We have adopted the terminology presented by Dixon and Rollin (2014). More precisely, firms with growth lower than the 10th quantile are considered to be rapidly shrinking firms, firms with zero growth are considered to be non-growing firms, and firms with growth higher than the 90th quantile are considered to be high-growth or rapidly growing firms.
Before presenting the quantile regression estimates, we first analyzed and compared variables for each group. This comparison among groups could indicate what drives SME growth. The econometric results, presented in Section 5, will show if those drivers still explain firm growth after controlling for several different factors.
In the sample, there are 867 rapidly shrinking firms, 2,101 non-growing firms and 811 high-growth firms. Table 6 presents the mean of the variables for each group.
Variable | Rapidly Shrinking Firms | Non-Growing Firms | High-Growth Firms |
---|---|---|---|
Past firm growth | 0.07 (0.30) | −0.004 (0.17) | −0.001 (0.28) |
Firm age | 21.77 (17.49) | 22.82 (19.27) | 18.95 (17.18) |
Firm size | 15.76 (33.79) | 6.13 (12.43) | 11.43 (26.27) |
Sales growth | −0.64 (2.41) | −0.53 (2.50) | −0.25 (2.28) |
Export (%) | 16.15 | 13.61 | 16.28 |
Expected export (%) | 15.34 | 12.47 | 20.47 |
Research and development (%) | 10.84 | 8.23 | 16.03 |
Machinery and equipment (%) | 39.22 | 37.84 | 46.24 |
Information and communication technology (%) | 40.95 | 39.03 | 45.75 |
No innovation (%) | 61.13 | 62.73 | 56.10 |
Innovation (products, processes or other) (%) | 26.76 | 28.80 | 35.76 |
Innovation (only organizational methods or new way of selling goods or services) (%) | 9.11 | 8.47 | 8.14 |
Training (%) | 39.22 | 31.27 | 39.46 |
Capital per employee ($) | 120,821.90 (384,917.50) | 153,139.00 (478,663.00) | 217,244.20 (861,624.60 ) |
Wages ($) | 37,695.00 (24,452.82) | 45,574.01 (81,400.10) | 45,997.85 (44,246.88 ) |
Debt ratio | 0.58 (0.82) | 0.51 (1.02) | 0.52 (0.64) |
Liquidity | 0.58 (0.30) | 0.60 (0.30) | 0.58 (0.29) |
Return on assets | 0.04 (0.46) | 0.13 (0.74) | 0.12 (0.37) |
Owner age | 52.46 (10.58) | 52.74 (10.76) | 50.51 (11.28) |
Education (%) | 29.30 | 31.41 | 33.05 |
Motivation (%) | 63.44 | 68.82 | 76.08 |
Indigenous Peoples (%) | 1.73 | 1.48 | 2.71 |
Visible minority (%) | 6.11 | 6.66 | 6.78 |
Immigrant status (%) | 15.69 | 15.99 | 17.76 |
Majority-female ownership (%) | 11.30 | 11.38 | 9.62 |
Number of observations | 867 | 2,101 | 811 |
Note: Standard deviation is in parentheses for continuous variables. For dummy variables, the proportion is reported instead of the mean.
Sources: Statistics Canada, Survey on Financing and Growth of Small and Medium Enterprises, 2011; General Index of Financial Information 20082014; and authors' calculations.
Table 6 suggests some firm characteristics as key drivers of SME growth. Rapidly shrinking firms have undergone a significantlyFootnote 31 higher growth rate, in terms of past firm size (7 percent), compared with non-growing firms (−0.4 percent) and high-growth firms (−0.1 percent). Past higher growth rates could be an indication that a firm will not grow at the same pace or will even shrink during the next years. Rapidly growing firms are younger than firms that diminished in size or remained the same size. On average, rapidly shrinking firms are 22 years old, non-growing firms are 23 years old and high-growth firms are 19 years old. Firm size is also significantly different among the three groups as high-growth firms are smaller than rapidly shrinking firms. On average, rapidly shrinking firms have 16 employees compared with 11 employees for high-growth firms. Non-growing firms are the smallest, with six employees on average.
Exporting goods or services does not seem to be a key driver of firm growth as the difference in the proportion of firms that export between rapidly shrinking firms and high-growth firms is not statistically different (around 16 percent). However, rapidly growing firms have a larger proportion of firms that invested in R&D, M&E and ICT compared with rapidly shrinking firms and firms that did not grow. Around 16 percent of high-growth firms invest in R&D compared with 11 percent of rapidly shrinking firms and 8 percent of non-growing firms. Forty-six percent of rapidly growing firms spent on new M&E in 2011 compared with 39 percent of rapidly shrinking firms and 38 percent of non-growing firms. Approximately 46 percent of high-growth firms spent on new ICT in comparison with 41 percent and 39 percent of rapidly shrinking firms and non-growing firms respectively. In addition, a significantly greater proportion (36 percent) of rapidly growing firms developed or introduced new or significantly improved goods or services, or a production process or method compared with rapidly shrinking firms (27 percent).
On average, capital and wages by employee are higher for rapidly growing firms than rapidly shrinking firms. Rapidly growing firms have $217,244 capital per employee and rapidly shrinking firms have around $120,822. In terms of wages paid, HGFs pay $45,998 per employee compared with $37,695 per employee by rapidly shrinking firms.
For firm performance indicators, we found a higher debt ratio for rapidly shrinking firms (0.58) compared with 0.51 and 0.52 for non-growing and high-growth firms respectively. Return on assets is significantly lower for rapidly shrinking firms (0.04) compared with non-growing firms (0.13) and high-growth firms (0.12).
Owner characteristics may play an important role in distinguishing firms that are at the top of the growth distribution from those at the bottom. Rapidly growing firms have a higher proportion of business owners with at least a university degree, about 33 percent. In comparison, 29 percent of rapidly shrinking firms have owners with at least a university degree. Owner motivation to grow is another key driver of SME growth. Here, the difference between high-growth firms, non-growing firms and rapidly shrinking firms is substantial. Approximately 76 percent of owners of high-growth firms expect yearly growth in sales or revenue of 10 percent or higher over the next 3 years. Conversely, only 63 percent of owners of rapidly shrinking firms and 68 percent of owners of non-growing firms expect yearly growth in sales or revenue of 10 percent or higher over the next 3 years.
4.7 Firm growth dynamics
This section investigates firm growth dynamics or, more precisely, firm growth processes. In other words, we want to assess if past firm growth is a guarantee of future firm growth. The literature often refers to this process as firm growth rate autocorrelation or firm growth persistence. Previous empirical results on the sign of firm growth autocorrelation are mixed and there is no clear consensus among the findings (Coad, 2007b). Positive autocorrelation means that past positive growth rates explainFootnote 32 positive current or future growth and that negative growth rates explain negative future growth. Negative autocorrelation means that past positive rates explain negative future growth and that past negative rates explain positive future growth. Some studies have shown positive autocorrelation of firm growth rates (Wagner, 1992; Bottazzi et al., 2001; Bottazzi and Secchi, 2003c), while others have shown negative autocorrelation (Bottazzi et al., 2007; Bottazzi et al., 2011). Finally, some researchers found no relationship between past and future firm growth (Bottazzi et al., 2002).
A possible explanationFootnote 33 of the divergence of results concerning autocorrelation of firm growth rates was provided by Coad (2007b). He found evidence that autocorrelation varies with firm size. He observed that small firms are subject to negative correlation and larger firms display positive correlation. Coad and Hölzl (2009) obtained similar results.
Firm growth persistence is particularly interesting for HGFs as economists and policy-makers want to know if firms that have undergone fast growth are likely to repeat the performance. As mentioned by Daunfeldt and Halvarsson (2015), however, there are only a few studies on persistence of rapid firm growth. In general, the authors noticed that rapidly growing firms are likely to be "one-hit wonders." Some authors have observed that firms that experienced rapid growth were more likely to repeat the experience and reach fast growth rates during a subsequent period (López-García and Puente, 2012; Rivard, 2020). Interestingly, Coad (2007b) also observed that small firms that undergo extreme positive or negative growth are unlikely to repeat the experience in the next year.
Table 7 presents a transition matrix for firm growth. We considered firm growth patterns by creating growth categoriesFootnote 34 and allocating firms using their past growth (20082011) versus their future growth (20112013).
Firm growth, 2011–2013 | |||||||
---|---|---|---|---|---|---|---|
< 0 | [0, 1) | [1, 10) | [10, 20) | ≥ 20 | Total | ||
Firm growth, 2008–2011 | < 0 | 34.59 | 26.76 | 7.65 | 8.23 | 22.77 | 2,235 |
[0, 1) | 21.72 | 51.57 | 2.98 | 3.95 | 19.77 | 1,846 | |
[1, 10) | 39.64 | 17.62 | 11.80 | 12.71 | 18.23 | 1,322 | |
[10, 20) | 40.74 | 18.09 | 8.97 | 11.25 | 20.94 | 702 | |
20 | 35.92 | 23.09 | 3.42 | 7.30 | 30.27 | 849 | |
Total | 2,289 | 2,106 | 474 | 566 | 1,519 | 6,954 |
Sources: Statistics Canada, Survey on Financing and Growth of Small and Medium Enterprises, 2011; General Index of Financial Information 20082014; and authors' calculations.
According to Contoyannis et al. (2004), the diagonal elements of the transition matrix are good indicators of firm growth persistence for the extreme categories. In Table 7, around 35 percent of firms that have undergone negative growth over the period 20082011 also withstood negative growth between 2011 and 2013. At the other extreme, 30 percent of firms that had a growth rate higher or equal to 20 percent between 2008 and 2011 exhibited the same performance over the period 20112013. In both cases, it shows some persistence.
Around half of the firms that had growth rates between 0 and 1 percent were in the same firm growth category over the period 20112013. This indicates some sort of persistence into slow/non-growth for 50 percent of the firms. From a policy perspective, it could indicate that the focus should shift from HGFs to slow-growth firms (SGFs). At the very least, it means that SGFs, or the reasons for not growing, should be investigated. For the in-between categories (firm growth rates greater or equal to 1 percent and less than 20 percent), we observed that, in general, firms did not stay in the same categories (i.e., no persistence).
It is worth mentioning that around 23 percent of firms that underwent negative growth over the period 20082011 grew by 20 percent or more over the period 20112013. As a consequence, firms that were at the bottom in terms of firm growth are likely to reach the top during the next period. We also observed that approximately 36 percent of firms that experienced growth equal to or greater than 20 percent over the period 20082011 had negative growth over the period 20112013. This again shows that there is no persistence.
Table 8 presents the distribution of each column by firm growth category over the period 20082011. In general, the figures are similar for each category of firm growth over the period 20112013 and follow the overall distribution of firms by category of firm growth between 2008 and 2011 (the last column of Table 8). Those numbers are shown in parentheses.
Firm growth, 2011–2013 | |||||||
---|---|---|---|---|---|---|---|
< 0 | [0, 1) | [1, 10) | [10, 20) | ≥ 20 | Total | ||
Firm growth, 2008–2011 | < 0 | 33.77 | 28.40 | 36.07 | 32.50 | 33.50 | 2,235 (32.14) |
[0, 1) | 17.52 | 45.20 | 11.61 | 12.88 | 24.03 | 1,846 (26.55) | |
[1, 10) | 22.89 | 11.06 | 32.91 | 29.69 | 15.87 | 1,322 (19.01) | |
[10, 20) | 12.49 | 6.03 | 13.28 | 13.95 | 9.68 | 702 (10.09) | |
20 | 13.32 | 9.31 | 6.13 | 10.95 | 16.92 | 849 (12.21) | |
Total | 2,289 | 2,106 | 474 | 566 | 1,519 | 6,954 |
Sources: Statistics Canada, Survey on Financing and Growth of Small and Medium Enterprises, 2011; General Index of Financial Information 20082014; and authors' calculations.
Table 8 reveals that the greatest contributor of each firm growth category (20112013) is firms that had negative growth between 2008 and 2011 (32 percent of firms in the sample had negative growth over the period 20082011). Thus, even for firms that experienced high growth (≥ 20) in 20112013, 33.5 percent experienced negative growth over the period 20082011, and only around 17 percent of firms would exhibit high-growth persistence.
5. Results
Table 9 presents estimates for seven quantile regressions ( = 0.05, 0.10, 0.25, 0.50, 0.75, 0.90, 0.95) and the ordinary least squares regression for comparison. All determinants considered in this study are included and fall into four categories: firm characteristics, barriers to growth, owner characteristics and macroeconomic factors. We will focus on the first three categories as the variables related to the fourth category (population growth and difference in unemployment rate, both considered by census metropolitan area) were not significant in the models. Also, we used the inverse probability weighting estimator,Footnote 35 as mentioned in Section 4.3.
Estimated coefficient interpretations in the context of quantile regressions are similar to OLS interpretations. An estimated coefficient can be interpreted as the marginal change in the dependent variable at the th conditional quantile caused by a small change in a chosen explanatory variable x (Coad et al., 2016). Following Cameron and Trivedi (2010), if we denote the quantile regression by , we have
Therefore, the marginal effect of the variable is
However, we assume that the observation stays in the same quantile of the distribution with a small change in the explanatory variable.
Variables | OLS | QR05 | QR10 | QR25 | QR50 | QR75 | QR90 | QR95 |
---|---|---|---|---|---|---|---|---|
Firm characteristics | ||||||||
Past firm growth | −0.192Footnote *** (0.050) | −0.267Footnote *** (0.039) | −0.307Footnote *** (0.046) | −0.148Footnote *** (0.029) | 0.001 (0.003) | −0.024Footnote * (0.013) | −0.015 (0.030) | −0.067 (0.054) |
Firm age | −0.012 (0.008) | 0.044Footnote *** (0.013) | 0.022Footnote ** (0.010) | 0.006 (0.005) | −0.001 (0.001) | −0.034Footnote *** (0.005) | −0.052Footnote *** (0.006) | −0.059Footnote *** (0.012) |
Firm size | −0.135Footnote *** (0.015) | −0.178Footnote *** (0.025) | −0.103Footnote *** (0.019) | −0.100Footnote *** (0.008) | −0.003table note * (0.002) | −0.095Footnote *** (0.020) | −0.254Footnote *** (0.015) | −0.281Footnote *** (0.022) |
Firm size squared | 0.019Footnote ** (0.003) | 0.034Footnote ** (0.005) | 0.021Footnote ** (0.003) | 0.016Footnote *** (0.002) | 0.001table note * (0.001) | 0.012Footnote *** (0.003) | 0.033Footnote *** (0.003) | 0.035Footnote *** (0.004) |
Sales growth | 0.009Footnote *** (0.003) | 0.009 (0.007) | 0.011Footnote ** (0.004) | 0.010Footnote ** (0.002) | 0.000 (0.000) | 0.006Footnote *** (0.002) | 0.008table note * (0.005) | 0.012Footnote *** (0.004) |
Export | −0.015 (0.015) | 0.008 (0.031) | −0.027 (0.019) | −0.010 (0.012) | 0.000 (0.002) | −0.016table note * (0.009) | 0.001 (0.016) | 0.01 (0.025) |
Expected export | 0.027 (0.016) | 0.012 (0.030) | −0.017 (0.023) | 0.001 (0.010) | 0.001 (0.002) | 0.049Footnote *** (0.009) | 0.060Footnote ** (0.023) | 0.044Footnote ** (0.022) |
Research and development | 0.015 (0.016) | −0.039 (0.037) | −0.022 (0.020) | 0.000 (0.012) | 0.001 (0.003) | 0.018table note * (0.010) | 0.047Footnote ** (0.018) | 0.064Footnote *** (0.022) |
Machinery and equipment | 0.027Footnote ** (0.011) | 0.042table note * (0.020) | 0.015 (0.016) | 0.031Footnote *** (0.008) | 0.001 (0.001) | 0.015Footnote ** (0.007) | 0.019table note * (0.010) | 0.036Footnote ** (0.017) |
Information and communication technology | 0.012 (0.011) | 0.047Footnote ** (0.021) | 0.017 (0.017) | −0.001 (0.008) | 0.000 (0.001) | 0.012table note * (0.007) | 0.004 (0.010) | −0.002 (0.017) |
Innovation Type 1 | 0.031Footnote ** (0.013) | −0.035 (0.023) | 0.010 (0.016) | 0.008 (0.008) | 0.001 (0.001) | 0.022Footnote *** (0.007) | 0.045Footnote *** (0.011) | 0.064Footnote *** (0.018) |
Innovation Type 2 | −0.0012 (0.020) | 0.0136 (0.042) | 0.0275 (0.024) | 0.0148 (0.011) | −0.0004 (0.002) | 0.0157 (0.015) | 0.0286Footnote ** (0.014) | 0.0305 (0.028) |
Training | 0.029Footnote ** (0.012) | 0.099Footnote *** (0.023) | 0.080Footnote *** (0.018) | 0.026Footnote *** (0.009) | 0.001 (0.001) | 0.015Footnote ** (0.007) | 0.019table note * (0.010) | 0.009 (0.020) |
Capital | 0.006table note * (0.003) | 0.024Footnote *** (0.005) | 0.020Footnote *** (0.007) | 0.007Footnote *** (0.002) | 0.000 (0.000) | 0.000 (0.002) | −0.002 (0.002) | −0.008 (0.005) |
Capital growth | −0.038Footnote *** (0.012) | −0.027Footnote *** (0.010) | −0.031Footnote ** (0.012) | −0.027Footnote *** (0.006) | −0.012Footnote *** (0.003) | −0.069Footnote *** (0.005) | −0.061Footnote *** (0.006) | −0.057Footnote *** (0.010) |
Wages | 0.049Footnote *** (0.013) | 0.096Footnote *** (0.015) | 0.072Footnote *** (0.017) | 0.045Footnote *** (0.007) | 0.001 (0.001) | 0.006 (0.009) | 0.001 (0.011) | −0.002 (0.015) |
Wage growth | −0.048Footnote ** (0.020) | −0.022 (0.023) | −0.083Footnote ** (0.035) | −0.050Footnote *** (0.015) | −0.003 (0.003) | −0.101Footnote *** (0.015) | −0.089Footnote *** (0.017) | −0.102Footnote *** (0.022) |
Debt ratio | −0.015Footnote ** (0.008) | −0.078Footnote *** (0.013) | −0.070Footnote ** (0.036) | −0.010 (0.023) | 0.000 (0.001) | −0.005 (0.006) | −0.017Footnote *** (0.005) | −0.026Footnote ** (0.012) |
Liquidity | −0.004 (0.024) | 0.130Footnote *** (0.039) | 0.106Footnote *** (0.038) | 0.020 (0.017) | 0.000 (0.002) | −0.004 (0.015) | −0.009 (0.021) | −0.099Footnote ** (0.039) |
Return on assets | 0.019 (0.013) | 0.027Footnote ** (0.013) | 0.024 (0.016) | 0.018 (0.012) | 0.000 (0.001) | 0.020 (0.018) | 0.030Footnote *** (0.010) | 0.029 (0.025) |
Barriers to growth | ||||||||
Shortage of labour | 0.025table note * (0.014) | −0.004 (0.025) | 0.011 (0.018) | 0.022Footnote ** (0.009) | 0.001 (0.001) | 0.036Footnote *** (0.008) | 0.029Footnote *** (0.010) | 0.008 (0.021) |
Fluctuations in demand for products or services | −0.026Footnote ** (0.011) | −0.023 (0.021) | −0.023 (0.016) | −0.022Footnote *** (0.008) | −0.001 (0.001) | −0.018Footnote ** (0.007) | −0.023Footnote ** (0.010) | −0.041Footnote ** (0.016) |
Obtaining financing | −0.006 (0.017) | −0.018 (0.032) | −0.037Footnote ** (0.018) | −0.021 (0.013) | 0.000 (0.001) | 0.014 (0.008) | 0.047Footnote *** (0.017) | 0.042Footnote ** (0.020) |
Government regulations | 0.000 (0.011) | −0.037table note * (0.022) | 0.000 (0.017) | 0.011 (0.008) | 0.000 (0.001) | −0.007 (0.007) | −0.011 (0.010) | 0.007 (0.017) |
Rising cost of inputs | 0.012 (0.012) | 0.044Footnote ** (0.021) | 0.019 (0.019) | 0.007 (0.008) | 0.000 (0.001) | −0.006 (0.007) | −0.021table note * (0.011) | −0.022 (0.018) |
Increasing competition | −0.020table note * (0.011) | −0.026 (0.021) | −0.026table note * (0.016) | −0.018Footnote ** (0.008) | −0.001 (0.001) | −0.012table note * (0.007) | −0.032Footnote *** (0.010) | −0.027 (0.017) |
Other external obstacles | 0.008 (0.013) | 0.013 (0.024) | 0.029table note * (0.017) | 0.010 (0.009) | 0.000 (0.001) | 0.010 (0.008) | 0.005 (0.013) | 0.038 (0.023) |
Managing debt level | 0.006 (0.018) | 0.007 (0.029) | −0.025 (0.022) | −0.024table note * (0.013) | 0.000 (0.001) | 0.008 (0.011) | 0.002 (0.014) | 0.000 (0.024) |
Maintaining sufficient cash flow | −0.009 (0.013) | −0.030 (0.025) | −0.013 (0.017) | 0.003 (0.010) | 0.000 (0.001) | −0.008 (0.008) | 0.004 (0.013) | 0.006 (0.021) |
Lack of monitoring business operations to make improvements | −0.031Footnote ** (0.016) | −0.064table note * (0.035) | −0.048Footnote *** (0.016) | −0.026Footnote ** (0.012) | −0.001 (0.002) | −0.010 (0.008) | 0.009 (0.014) | 0.024 (0.019) |
Lack of knowledge about competitors or market trends | 0.005 (0.017) | −0.013 (0.027) | 0.003 (0.025) | 0.011 (0.014) | 0.000 (0.001) | −0.015 (0.009) | 0.016 (0.014) | 0.012 (0.025) |
Devoting too much time to day-to-day operations | −0.008 (0.011) | 0.049Footnote ** (0.021) | 0.018 (0.016) | 0.00 (0.008) | 0.000 (0.001) | −0.011 (0.007) | −0.026Footnote *** (0.010) | −0.075Footnote *** (0.017) |
Recruiting and retaining employees | 0.028Footnote ** (0.014) | 0.040 (0.026) | 0.020 (0.018) | 0.00 (0.009) | 0.001 (0.001) | 0.015Footnote ** (0.008) | 0.035Footnote *** (0.011) | 0.066Footnote *** (0.021) |
Other internal obstacles | 0.010 (0.019) | −0.082 (0.052) | 0.033 (0.029) | 0.00 (0.013) | 0.000 (0.002) | −0.014 (0.011) | 0.012 (0.026) | 0.052 (0.034) |
Owner characteristics | ||||||||
Age | −0.081Footnote *** (0.027) | −0.116Footnote ** (0.047) | −0.104Footnote *** (0.035) | −0.01 (0.020) | −0.002 (0.002) | −0.116Footnote *** (0.019) | −0.110Footnote *** (0.024) | −0.112Footnote *** (0.042) |
Education | 0.033Footnote *** (0.013) | 0.077Footnote *** (0.023) | 0.072Footnote *** (0.017) | 0.029Footnote ** (0.008) | 0.000 (0.001) | 0.010 (0.007) | 0.021table note * (0.012) | 0.060Footnote *** (0.018) |
Motivation | 0.088Footnote *** (0.013) | 0.212Footnote *** (0.025) | 0.193Footnote *** (0.022) | 0.084Footnote *** (0.014) | 0.002Footnote ** (0.001) | 0.070Footnote *** (0.007) | 0.029Footnote ** (0.013) | 0.030 (0.020) |
Indigenous Peoples | 0.034 (0.037) | 0.173Footnote *** (0.060) | 0.008 (0.067) | −0.027table note * (0.015) | 0.001 (0.008) | 0.014 (0.044) | 0.078 (0.062) | 0.050 (0.046) |
Visible minority | −0.029 (0.025) | 0.045 (0.046) | −0.039 (0.035) | −0.025 (0.020) | −0.001 (0.002) | −0.046Footnote *** (0.014) | −0.045 (0.033) | −0.075table note * (0.043) |
Immigrant status | 0.011 (0.018) | −0.016 (0.034) | 0.000 (0.021) | 0.005 (0.013) | 0.000 (0.001) | 0.018table note * (0.010) | 0.011 (0.014) | 0.077Footnote ** (0.036) |
Majority-female ownership | −0.024 (0.018) | −0.050Footnote ** (0.025) | −0.046 (0.031) | −0.034Footnote ** (0.016) | −0.001 (0.001) | −0.024Footnote ** (0.012) | −0.026 (0.017) | −0.038 (0.026) |
Macroeconomic factors | ||||||||
Population growth | 1.019 (1.047) | -2.832 (1.949) | 0.882 (1.528) | −0.002 (0.775) | 0.001 (0.085) | 2.009Footnote *** (0.604) | 2.397Footnote ** (0.939) | 2.704 (1.879) |
Unemployment rate growth | 0.018 (0.023) | 0.028 (0.042) | 0.002 (0.032) | 0.021 (0.017) | 0.001 (0.002) | −0.02 (0.014) | 0.00 (0.021) | 0.052 (0.040) |
Constant | −0.102 (0.168) | -1.546Footnote *** (0.251) | -1.058Footnote *** (0.218) | −0.566Footnote *** (0.114) | −0.004 (0.014) | 0.712Footnote ** (0.115) | 1.233Footnote *** (0.150) | 1.602Footnote *** (0.218) |
Pseudo-R2 | 0.079 | 0.085 | 0.097 | 0.054 | 0.000 | 0.056 | 0.113 | 0.130 |
Number of observations | 6,954 | 6,954 | 6,954 | 6,954 | 6,954 | 6,954 | 6,954 | 6,954 |
Note 3: Coefficients for the region and sector dummy variables are controlled for, but not reported.
Note 4: For quantile regressions, pseudo-R2 is calculated as one minus the sum of weighted deviations about the estimated quantile divided by the sum of weighted deviations about the raw quantile (StataCorp, 2015).
Note 5: Quantile regressions and the OLS regression are estimated using the inverse probability weighting estimator to adjust for selection (the option pweights in Stata).
Sources: Statistics Canada, Survey on Financing and Growth of Small and Medium Enterprises, 2011; General Index of Financial Information 2008–2014; and authors' calculations.
Table 9, as well as Figures 4 and 5, show the estimated coefficients for quantile regressions and the OLS estimate for some of the main variables of the model.Footnote 36 Looking at the figures, it is possible to visualize how the estimated coefficients by quantile regression differ from the estimated coefficients by OLS. Dashed lines are the estimated coefficients by OLS and the continuous lines are the estimated coefficients from quantile regressions. It is obvious from these figures (as well as from Table 9) that the impact of the variables on firm growth varies greatly relative to where firms are located on the distribution. Clearly, the relationship between explanatory variables and firm growth is non-linear.
Sections 5.1 to 5.3 will present the interpretations of estimated coefficients (i.e., explanatory variables) on firm growth for firms that experienced a substantial increase or decrease in employment, i.e., firms on the right-hand side of the firm growth distribution (i.e., 90th and 95th quantiles) and firms on the left-hand side of the firm growth distribution (i.e., 5th and 10th quantiles). As mentioned in Section 4.6, the former firms are commonly referred to as rapidly growing firms or HGFs and the latter as rapidly shrinking firms (Dixon and Rollin, 2014). For this study, we are particularly interested in the extreme of the conditional firm growth distribution and that is why we restricted our analysis to explanatory variables that affect the lower and upper tails of the distribution.
5.1 Firm characteristics
Table 9 shows that the relationship between firm growth and firm size is non-linear. For all quantile regressions, the estimated coefficient of firm size is negative, with a positive estimated coefficient for the quadratic term — both are statistically significant. This shows that the relationship between firm growth and firm size takes the form of a U-shaped curve. Therefore, firm size has a negative impact on firm growth until the firm's size reaches a certain threshold, and firm size has a positive impact on firm growth thereafter. This result could be explained by the fact that smaller firms (e.g., 14 employees) tend to stay small over time (Archambault and Song, 2018). This finding is in line with what we mentioned in Section 4.6, i.e., non-growing firms are smaller, on average, with around six employees (Table 6). Firms start to grow when they reach a specific threshold size. As a consequence, the effect of firm size on firm growth is positive. Similar results have been observed in the literature: Evans (1987), Dunne et al. (1989), Liu et al. (1999), Goedhuys and Sleuwaegen (2010), Park et al. (2010), Schoonjans et al. (2013) and Chang and Lin (2017). Another consequence is that Gibrat's law does not hold for Canadian SMEs as firm growth and firm size are not independent.
To determine if the overall effect of firm size is positive or negative, we calculated the marginal effect of firm size on firm growth (Schoonjans et al., 2013) and, in particular, the average partial effects.Footnote 37 For all of the quantile regressions and the OLS regression, a negative relationship between firm size and firm growth was observed. This result has been largely noticed in the literature and could be interpreted as follows: smaller firms grow at a faster pace than larger firms (Goedhuys and Sleuwaegen, 2010). The magnitude of average partial effects is greater for rapidly growing firms (Figure 3), which means that the impact of firm size is more negative on firms that undergo higher growth.
Figure 3: Average partial effects (APE) of firm size on firm growth
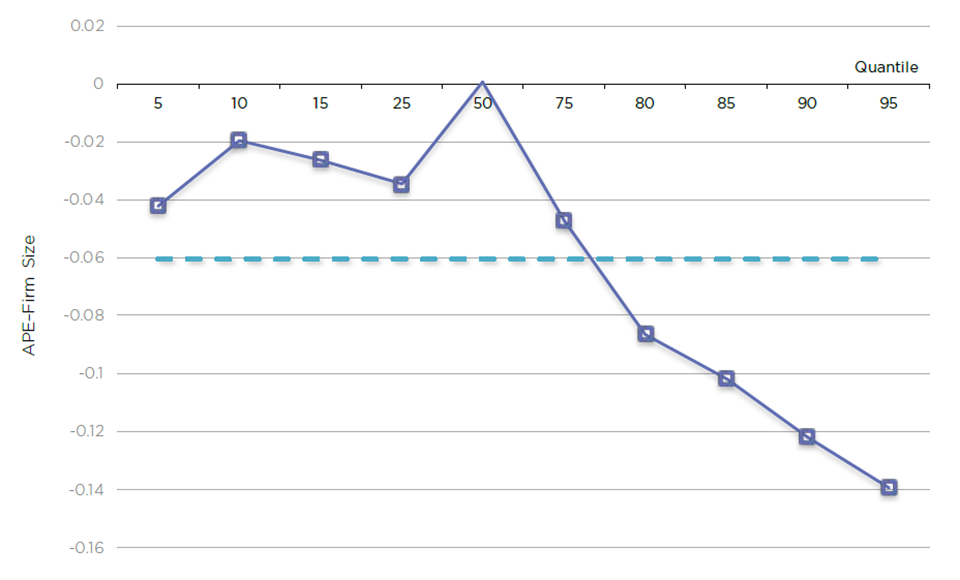
Note 2: Estimated average partial effects significant at least at 10 percent are indicated by a "".
Sources: Statistics Canada, Survey on Financing and Growth of Small and Medium Enterprises, 2011; General Index of Financial Information 2008–2014; and authors' calculations.
For firm age, Figure 4 reveals that age is positively related to growth for firms that experienced a decrease in size (negative growth), but is negatively related to growth for firms that experienced an important increase in size. We also added into the model a variable related to a firm's past growth to control for serial correlation. The relationship between past firm growth and the current period is negative and significant for rapidly shrinking firms.
Sales growth also has a positive impact on firm growth and its magnitude is similar across the 5th, 10th, 25th, 75th, 90th and 95th quantiles.
Another variable of interest is the dummy variable related to export in 2011. However, the variable is not significantly different from zero for most of the quantile regressions. The result obtained does not show strong evidence that the export variable has a positive impact on firm growth when employment is used as a measure of firm size.Footnote 38 The variable "expected export," related to the owner's intention to expand sales to new markets outside of Canada, is positively related to firm growth for firms that undergo positive growth. In particular, businesses that expect to expand to new markets see an increase in firm size by four to six percentage points. Caution should be taken in this case as the variable expressed only an intention to expand to new markets and we do not know for sure if the business actually exported after 2011.
Investment in new information and communication technology has a positive and significant impact on firm growth for firms in the 5th quantile that experienced rapid shrinking, increasing firm growth by approximately five percentage points. However, there is no clear evidence that investment in information and communication technology has a strong overall impact on firm growth as the coefficients obtained were not significant for several quantiles.
Investment in R&D and innovation reveals positive and significant repercussions on firm growth, especially for firms that underwent a substantial increase in employment. Also, the impact is stronger when firm growth is greater — the estimated coefficients increase continuously for the upper quantiles. At the 95th quantile, firms that spend on R&D increase their growth by around six percentage points.Footnote 39 On the other hand, the impact of R&D expenditures is not significantly different from zero for firms that are diminishing in size. Falk (2012) obtained similar results. Investment in M&E is likely another driver of firm growth as it had a positive and significant impact on firm employment growth. For the highest growth quantiles (90th and 95th), investment in M&E increased firm growth by almost two to four percentage points. For lower quantiles, where firms are rapidly shrinking, investment in M&E was also non-negligible as it helped to avoid the decrease in growth by four percentage points.
Development or introduction of a new or improved good or service, or a new and significantly improved production process or method, over the past 3 years (20092011), seems to have a notable impact on rapidly growing firms. Innovative firms at the 90th and 95th quantiles increased their growth by four and six percentage points, respectively, compared with those in the same quantiles that did not innovate. Businesses that developed or introduced only a new organizational method or a new way of selling goods or services process over the past 3 years and no other type of innovation (innovation type 2) experienced a positive and significant impact on growth at the 90th quantile, improving growth by around three percentage points.
Expenditures on education and training for employees, which could be seen as an investment in human capital, seem to have a positive influence on firm growth. In particular, the impact seems more important for firms that have diminished in size as it increased their growth by eight to nine percentage points.
Capital has a positive and significant impact on firm growth, especially for those firms that have undergone a great reduction in size. The estimated coefficient is not significant for quantiles higher than the 50th quantile. Capital growth is significant, but its relationship to firm growth is negative. From the firm's production perspective, this makes sense as there is generally substitution between capital and labour.
The relationship between firm growth and wage level is positive, but significant only for the 5th, 10th and 25th quantiles. For other quantiles, the impact is near zero and non-significant. Within this context, the level of wages could be seen as a proxy for human capital and may have a positive impact on firms experiencing a considerable decrease in size. On the other hand, wage growth has a negative effect on firm growth, and the estimated coefficient is significant for all quantiles except the 5th and 50th quantiles. In this case, wages are related to labour costs, which may hamper firm growth.
The model contains various firm performance indicators and they have different impacts on firm growth. A high level of current debt compared with total assets (debt ratio) has a negative impact on firm growth, particularly for those firms that have undergone a substantial decline in employment. The impact is also negative, but to a lesser extent, for firms that have increased in size. The relationship between liquidity (cash flow) and firm growth is positive and significant for firms that have decreased in size. The relationship is negative for firms that have increased in size, but it is significant only for the 95th quantile.
We observed that ROA, which is another firm performance indicator related to firm profitability, is not significant for most quantiles.
5.2 Barriers to growth
An analysis of internal and external obstacles to growth mentioned by firm owners or general managers in 2011 reveals some interesting facts on their impact on firm growth 2 years later (Table 9). In particular, for external obstacles, fluctuations in demand for products or services had a negative impact on firm growth, particularly for businesses that experienced an important increase in size. Difficulty in obtaining financing seems to have had a positive impact on firm growth. Regarding internal obstacles to business growth, three obstacles are worth mentioning. The first is a lack of monitoring business operations to make improvements. This had a negative impact on firm growth, especially for those firms that underwent a considerable decrease in employment. The second is devoting too much time to day-to-day operations. The relationship between firm growth and this obstacle is negative, mainly for firms that greatly increased in size over the period 20112013. The third is recruiting and retaining employees. This obstacle had a positive and significant effect on firm growth for firms that had an important increase in employment size. This result seems to be contradictory, but may be explained by the lag between the year when this obstacle was observed and the firm's growth (measured 2 years later). Another explanation could be that the owner (or general manager) took action and, as a result, increased the size of the business.
5.3 Owner characteristics
The model also contains variables related to characteristics of the owner. It is well known that personality traits of entrepreneurs could have an important influence on firm performance (Kerr et al., 2017). Figure 5 presents the results obtained for three owner characteristics: age, education and motivation. The relationship between firm growth and owner age is negative and significant for firms that have diminished in size and also for those firms that have increased in size — specifically, firms at the top of the distribution. Thus, younger entrepreneurs are more likely to be associated with rapidly growing firms.
Owners with a university degree seem to have a positive and significant impact on firm growth for all quantiles, except the 50th quantile. Moreover, the impact is greater for firms that had an important decrease in size compared with those that have had a large increase in size. This finding is similar to that observed by Goedhuys and Sleuwaegen (2010). At the 5th and 10th quantiles, having a university degree increased firm growth by eight and seven percentage points respectively. At the 90th and 95th quantiles, having a university degree increased firm growth by two and six percentage points respectively.
Figure 4: OLS and quantile regression estimates
Firm characteristics
Figure 4a: Estimated coefficient of Firm growth past
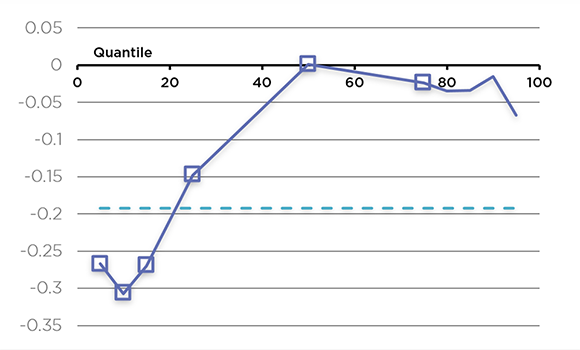
Figure 4b: Estimated coefficient of Firm age
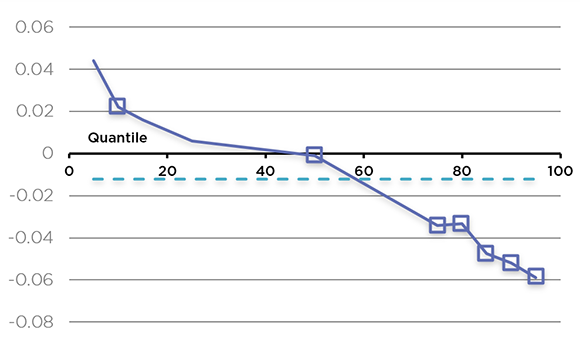
Figure 4c: Estimated coefficient of Sales Growth

Figure 4d: Estimated coefficient of R&D

Figure 4e: Estimated coefficient of M&E
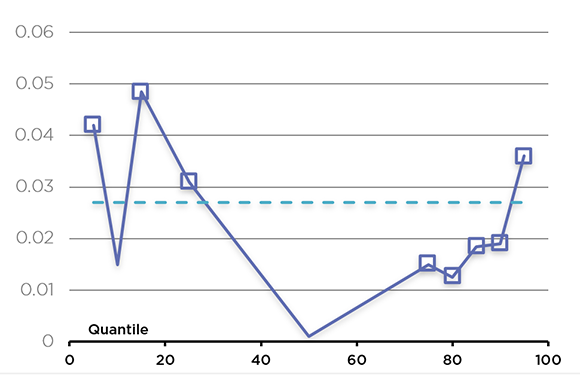
Figure 4f: Estimated coefficient of Innovation type 1
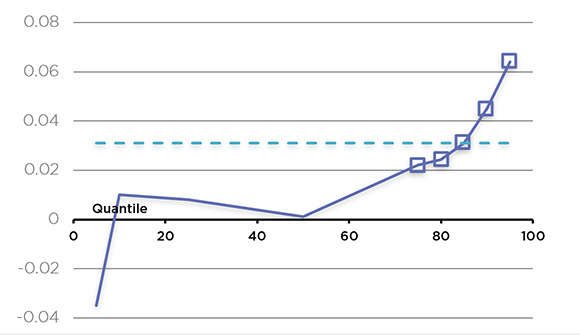
Figure 4g: Estimated coefficient of Training
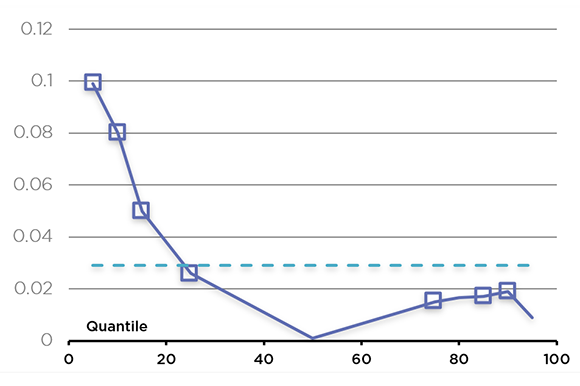
Figure 4h: Estimated coefficient of Capital level and growth
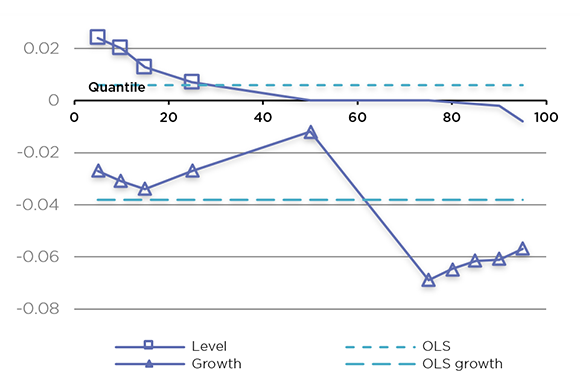
Note 1: The dashed line shows the estimated coefficient derived from OLS and the continuous line shows the estimated coefficients derived from quantile regressions.
Note 2: Estimated coefficients significant at least at 10 percent are indicated by a "" or a "" on each graph.
Sources: Statistics Canada, Survey on Financing and Growth of Small and Medium Enterprises, 2011; General Index of Financial Information 2008–2014; and authors' calculations.
Figure 5: OLS and quantile regression estimates, firm and owner characteristics
Figure 5a: Estimated coefficient of Wage level and growth

Figure 5b: Estimated coefficient of Debt ratio
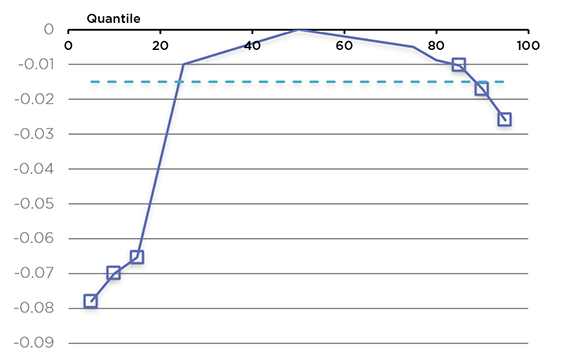
Figure 5c: Estimated coefficient of Liquidity

Figure 5d: Estimated coefficient of Owner age
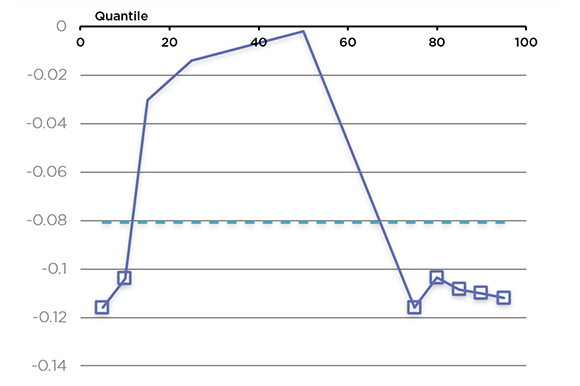
Figure 5e: Estimated coefficient of Education

Figure 5f: Estimated coefficient of Motivation

Note 1: The dashed line shows the estimated coefficient derived from OLS and the continuous line shows the estimated coefficients derived from quantile regressions.
Note 2: Estimated coefficients significant at least at 10 percent are indicated by a "" or a "" on each graph.
Sources: Statistics Canada, Survey on Financing and Growth of Small and Medium Enterprises, 2011; General Index of Financial Information 2008–2014; and authors' calculations.
Another potential driver of firm growth is what we defined as the motivation of the entrepreneur. It corresponds with the entrepreneur's intention to grow in terms of sales or total revenues over the next 3 years. The intention of the entrepreneur to grow is rarely captured in models relative to firm growth and could definitely be a fundamental internal determinant. However, as mentioned by Dobbs and Hamilton (2007), the intention to grow does not always convert into real growth. The Survey on Financing and Growth of Small and Medium Enterprises, 2011 has the advantage of having collected that information. Our results showed that a motivated owner has a positive impact on firm growth, particularly for rapidly shrinking firms. Motivation could increase firm growth by 19 to 21 percentage points for those firms. The potential impact is also significantly positive for rapidly growing firms, although the magnitude of the potential impact is smaller — owner motivation could increase firm growth by three to seven percentage points for firms in the 90th and 85th quantiles respectively.
Other characteristics related to the owner (or general manager) seem to have only limited significance on firm growth. We did not obtain consistent resultsFootnote 40 for majority-female ownership, visible minority, Indigenous peoples or immigrant status variables. Differences between majority-female ownership and majority-male ownership appear to be significant (at the 5 percent level) for only three quantile regressions (5th, 25th and 75th quantiles). Majority-female ownership seems to have a negative impact on firm growth, decreasing firm growth by five, three and two percentage points, respectively, for firms in the 5th, 25th and 75th quantiles. Overall, it is important to note that the findings show that there is no difference between majority-female and majority-male ownership in terms of drivers for rapidly growing firms as the variable on gender is not significantly different from zero for the upper quantiles. Even at the mean (OLS regression), the coefficient is not significantly different from zero.
Finally, this section does not contain a subsection on macroeconomic factors, which is the fourth category of explanatory variables involved in the regression models. The reason is simple. The variables considered — population growth and unemployment rate — were, in general, not significant for the quantile regressions. This contrasts with the results of Ipinnaiye et al. (2017), who observed a significant relationship between firm growth and unemployment rate, inflation and real effective exchange rate.
As in any research study, this analysis has limitations. Endogeneity is likely a potential issue and takes various forms: correlation between explanatory variables and error terms, sample selection, omitted explanatory variables, simultaneity, etc. Selection bias is a possible issue as firm growth is conditional on firm survival. The inverse probability weighting approach was used to overcome this issue by allowing more weight to firms that are more likely to exit. By doing this, we get a more representative sample. The omitted-variable bias is another possible issue. However, this could be unlikely as we controlled for variables that are related to different dimensions and determinants of firm growth, including internal factors (firm characteristics and owner characteristics) and external factors (macroeconomic variables). It should be noted that with these limitations, the empirical results obtained here may be related to conditional correlation between firm growth and the variables and not causal effects.
5.4 Robustness analysis
We tested the robustness of our findings by replicating the analysis at a disaggregated level by industry sector and firm size. We investigated the results for each industry sector and found heterogeneity among the sectors. Some variables that were strongly significant for the entire sample were not statistically significant when disaggregated at a sectoral level. As mentioned by Coad (2010), this could be explained by the high level of heterogeneity among firms. Also, the effects on growth might not have been totally captured by firms as some sectors contain only a few firms.
We also divided the sample in three categories: micro (1–4 employees), small (5–99 employees) and medium-sized firms (100 employees and more). Previous research has shown that the growth process varies largely between small and larger firms (Coad, 2007b, 2010). Overall, we obtained similar results, in terms of the estimated parameter sign and statistical significance, for micro and small firms as well as for the entire sample. One major difference is the non-linearity related to firm size. We obtained a U-shaped curve for micro firms and an inverted U-shaped curve for small firms. The average partial effect of firm size was negative for micro firms, but positive for small firms for most of the quantiles, except those at the top of the firm growth distribution. We also observed that owner's education and investment in M&E were non-significant for micro firms. For medium-sized firms, some of the effects were not statistically significant (e.g., firm age and investment in R&D, M&E and training). This could be explained, as mentioned earlier, by the fact that the observations did not capture all the effects due to the limited number of observations.
Finally, we tested the robustness of the results by changing the time frame. We considered firm growth over the period 20112014 and largely found the same results as for the period 20112013. The only exceptions were the variables related to investment in R&D and innovation. Those variables were not significant over the period 20112014. This result seems a bit counterintuitive as previous research argued that R&D investments and innovations generally have a lagged time effect on business growth. As the information on innovation concerns the period 20092011, however, it is conceivable that, for example, 2009 innovations had an impact on firm employment growth over the subsequent 4 years and started to fade after that. Lee et al. (2003) published results on the speed of innovation diffusion for specific sectors and type of product innovations. The average speed of innovation diffusion was 2.6 years and fluctuated with the industry considered as well as with the radicality and scope of innovation.
6. Conclusions
The main objective of this paper is to understand the factors that have an impact on firm growth, specifically on Canadian SMEs. The paper complements and enriches the empirical literature on the mechanism underlying firm growth. To do that, we used Statistics Canada's Survey on Financing and Growth of Small and Medium Enterprises, 2011 linked to administrative data, such as the General Index of Financial Information and Payroll Account Deductions and Remittances (PD7) from 20082014. For this report, we studied firm employment growth for the period 20112013. An investigation of firm growth rate distribution shows that it is tent shaped and heavier in the tails, with a greater density near zero than a Gaussian distribution.
Firm growth dynamics were also assessed by allocating firms using their past growth (20082011) versus their future growth (20112013). Firm growth shows some persistence, i.e., firms that had negative growth in the past or slow growth (growth rates between 0 and 1 percent) are likely to experience the same in the future. The same can be said for rapidly growing firms as 30 percent of firms that had a growth rate equal to or greater than 20 percent in 20082011 experienced the same in 20112013. However, firm growth dynamics are also volatile, e.g., firms that had negative growth in the past are likely to experience rapid growth in the future (23 percent). Also, firms that grew rapidly in the past are likely to shrink in the future (36 percent). Results reveal that firms that experienced negative growth in 20082011 are most likely (34 percent) to exhibit growth rates equal to or greater than 20 percent between 2011 and 2013. In comparison, rapidly growing firms only contributed to 17 percent of that category in 20112013.
We used the quantile regression technique, combined with inverse probability weighting, to analyze the key factors of firm growth. We controlled for firm characteristics, owner characteristics, barriers to growth and macroeconomic factors. The main contribution of this paper is to obtain empirical results from regressions where we controlled for various factors as firm growth derived from a complex multi-dimensional phenomenon. Covering such a large set of variables in the Canadian context is still rare in the firm growth literature.
Our empirical findings suggest asymmetric effects for rapidly shrinking firms, non-growing firms and rapidly growing firms. Overall, we found that most of the variables used in the quantile regressions have no correlation with growth for non-growing firms as the estimated coefficients are not significantly different from zero.
Past firm growth has a significant but negative impact on firms that are shrinking, but no impact on rapidly growing firms. Firm age has a positive impact on firms that are rapidly shrinking (10th quantile). It has a negative impact on high-growth firms, with the magnitude of the effects increasing (more negative) in the upper quantiles. We also found a non-linear relationship between firm size and firm growth, taking the form of a U-shaped curve, for all quantiles. By calculating the average partial effects, we found that the overall effect of firm size on firm growth is negative.
Investment in R&D, M&E and innovation (i.e., development or introduction of new or significantly improved goods or services, or a production process or method) has a positive and significant impact on rapidly growing firms, with the magnitude of the effect increasing in the upper quantiles. Investment in M&E has a positive and significant impact on firm growth for rapidly shrinking firms. Investment in employee training has a positive and significant impact on both rapidly shrinking firms and high-growth firms. Interestingly, the magnitude of the effect is greater on shrinking firms.
We also examined the impact of different financial variables on firm growth. Empirical results suggest that debt ratio has a significant and negative impact on firm growth, with a greater impact on rapidly shrinking firms. Liquidity is another important SME growth driver, particularly for rapidly shrinking firms as it has a positive and significant impact on firm growth.
With respect to owner characteristics, we found that owner age, education and motivation have significant impacts on firm growth. Owner age has a negative impact on rapidly shrinking firms and high-growth firms. Owners with university as the highest level of education attained positively influence firm growth for both rapidly shrinking and rapidly growing firms. Interestingly, the magnitude of the effect is greater in the lower quantiles. Finally, owner motivation to grow has a positive impact on firm growth, with the magnitude of the effect being greater for rapidly shrinking firms than high-growth firms.
To conclude, some policies may be derived from the empirical results obtained in this study. Evidence suggests that to play an active role on both two fronts, i.e., helping firms that are undergoing a strong decline in employment and firms that are growing rapidly, investment in M&E, training for employees and owner education are key factors to consider. Also, measures that foster investment in R&D, as well as helping firms to innovate, are particularly important for rapidly growing firms, and evidence suggests that this could be the impulse needed for firms to grow rapidly.
References
Acs, Z. J., Parsons, W., and Tracy, S. (2008). High-Impact Firms: Gazelles Revisited. Washington, D.C.: U.S. Small Business Administration, Office of Advocacy.
Archambault, R., and Song, M. (2018). Canadian New Firms: Birth and Survival Rates Over the Period 20022014. Ottawa: Innovation, Science and Economic Development Canada.
Ashton, T. S. (1926). The growth of textile businesses in the Oldham District, 18841924. Journal of the Royal Statistical Society, 89(3), 567583.
Audretsch, D. B., Klomp, L., Santarelli, E., and Thurik, A. R. (2004). Gibrat's law: Are the services different? Review of Industrial Organization, 24(3), 301324.
Barney, J.B. (1997). On Flipping Coins and Making Technology Choices: Luck as an Explanation of Technological Foresight and Oversight. In R. Garud, P. Nayyar, and Z. Shapira, eds., Technological Innovation: Oversight and Foresights (pp. 13–19). New York: Cambridge University Press.
Birch, D. (1979). The Job Generation Process. Cambridge: MIT Program on Neighborhood and Regional Change.
Bottazzi, G., and Secchi, A. (2003a). A stochastic model of firm growth. Physica A: Statistical Mechanics and its Applications, 324(1), 213219.
Bottazzi, G., and Secchi, A. (2003b). Why are distributions of firm growth rates tent-shaped? Economics Lettes, 80(3), 415420.
Bottazzi, G., and Secchi, A. (2003c). Common properties and sectoral specificities in the dynamics of U.S. manufacturing companies. Review of Industrial Organization, 23(3), 217232.
Bottazzi, G., and Secchi, A. (2005a). On the Laplace Distribution of Firm Growth Rates. Sant'Anna School of Advanced Studies. Laboratory of Economics and Management Working Paper Series, No. 2002/20.
Bottazzi, G., and Secchi, A. (2005b). Explaining the Distribution of Firms Growth Rates. Sant'Anna School of Advanced Studies. Laboratory of Economics and Management Working Paper Series, No. 2005/16.
Bottazzi, G., Dosi, G., Lippi, M., Pammolli, F., and Riccaboni, M. (2001). Innovation and corporate growth in the evolution of the drug industry. International Journal of Industrial Organization, 19(7), 11611187.
Bottazzi, G., Cefis, E., and Dosi, G. (2002). Corporate growth and industrial strutures: Some evidence from the Italian manufacturing industry. Industrial and Corporate Change, 11(4), 705723.
Bottazzi, G., Cefis, E., Dosi, G., and Secchi, A. (2007). Invariances and diversities in the patterns of industrial evolution: Some evidence from Italian manufacturing industries. Small Business Economics, 29(1), 137159.
Bottazzi, G., Coad, A., Jacoby, N., and Secchi, A. (2011). Corporate growth and industrial dynamics: Evidence from French manufacturing. Applied Economics, 43(1), 103116.
Calvino, F., Criscuolo, C., and Menon, C. (2016). No Country for Young Firms?: Start-Up Dynamics and National Policies. Paris: OECD Science, Technology and Industry Policy Papers, No. 29, OECD Publishing.
Cameron, A. C., and Trivedi, P. K. (2005). Microeconometrics: Methods and Applications. New York: Cambridge University Press.
Cameron, A. C., and Trivedi, P. K. (2010). Microeconometrics Using Stata (Vol. 2). College Station, Texas: Stata Press.
Capasso, M., Cefis, E., and Frenken, K. (2013). On the existence of persistently outperforming firms. Industrial and Corporate Change, 23(4), 9971036.
Chang, C.-F., and Lin, C.-S. (2017). The role of human capital in manufacturing plant growth: Evidence from Taiwan. Pacific Economic Review, 22(4), 554584.
Clayton, R. L., Sadeghi, A., Spletzer, J. R., and Talan, D. M. (2013). High-employment-growth firms: Defining and counting them. Monthly Labor Review, 136, 312.
Coad, A. (2007a). Firm Growth: A Survey. Documents de travail du Centre d'Economie de la Sorbonne 2007.24.
Coad, A. (2007b). A closer look at serial growth rate correlation. Review of Industrial Organization, 31(1), 6982.
Coad, A. (2010). Exploring the processes of firm growth: Evidence from a vector auto-regression. Industrial and Corporate Change, 19(6), 16771703.
Coad, A., and Hölzl, W. (2009). On the autocorrelation of growth rates. Journal of Industry, Competition and Trade, 9(2), 139166.
Coad, A., and Hölzl, W. (2012). Firm Growth: Empirical Analysis. In M. Dietrich and J. Krafft, eds., Handbook on the Economics and Theory of the Firm. Cheltenham, U.K.: Edward Edgar Publishing.
Coad, A., and Rao, R. (2008). Innovation and firm growth in high-tech sectors: A quantile regression approach. Research Policy, 37(4), 633648.
Coad, A., Daunfeldt, S.-O., Hölz, W., Johansson, D., and Nightingale, P. (2011). Who Do High-Growth Firms Employ, and Who Do They Hire? Ratio Working Papers, No. 169.
Coad, A., Daunfeldt, S.-O., Hölz, W., Johansson, D., and Nightingale, P. (2014). High-growth firms: Introduction to the special section. Industrial and Corporate Change, 23(1), 91–112.
Coad, A., Segarra, A., and Teruel, M. (2016). Innovation and firm growth: Does firm age play a role? Research Policy, 45(2), 387400.
Contoyannis, P., Jones, A. M., and Rice, N. (2004). The dynamics of health in the British Household Panel Survey. Journal of Applied Econometrics, 19(4), 473503.
Côté, S., and Rosa, J. M. (2017). Comparing Different Measures of High-Growth Enterprises: A Canadian Case Study. Ottawa: Innovation, Science and Economic Development Canada.
Daunfeldt, S.-O., and Halvarsson, D. (2015). Are high-growth firms one-hit wonders? Evidence from Sweden. Small Business Economics, 44(2), 361383.
Davidson, R., and MacKinnon, J. G. (2004). Econometric Theory and Methods. Oxford University Press .
Davidsson, P., and Henrekson, M. (2002). Determinants of the prevalence of start-ups and high-growth firms. Small Business Economics, 19(2), 81104.
Decker, R. A., Haltiwanger, J., Jarmin, R. S., and Miranda, J. (2016). Where has all the skewness gone? The decline in high-growth (young) firms in the U.S. European Economic Review, 86, 4–23.
Dixon, J., and Rollin, A.-M. (2014). The Distribution of Employment Growth Rates in Canada: The Role of High-Growth and Rapidly Shrinking Firms. Ottawa: Statistics Canada, Economic Analysis Division.
Dobbs, M., and Hamilton, R. T. (2007). Small business growth: Recent evidence and new directions. International Journal of Entrepreneurial Behaviour & Research, 13(5), 296322.
Du, J., and Temouri, Y. (2015). High-growth firms and productivity: Evidence from the United Kingdom. Small Business Economics, 44(1), 123143.
Dunne, T., Roberts, M. J., and Samuelson, L. (1989). The growth and failure of U.S. manufacturing plants. The Quarterly Journal of Economics, 104(4), 671698.
Erlingsson, E., Alfarano, S., Raberto, M., and Stefánsson, H. (2012). On the distributional properties of size, profit and growth of Icelandic firms. Journal of Economic Interaction and Coordination, 8(1), 5774.
EurostatOECD. (2007). Eurostat-OECD Manual on Business Demography Statistics. Luxembourg: Office for Official Publications of the European Communities.
Evans, D. S. (1987). Tests of alternative theories of firm growth. Journal of Political Economy, 95(4), 657674.
Falk, M. (2012). Quantile estimates of the impact of R&D intensity on firm performance. Small Business Economics, 39(1), 1937.
Fitzgerald, J., Gottschalk, P., and Moffitt, R. (1998). An analysis of sample attrition in panel data. Journal of Human Resources, 33(2), 251299.
Gibrat, R. (1931). Les inégalités économiques. Paris: Librairie du Recueil Sirey.
Goedhuys, M., and Sleuwaegen, L. (2010). High-growth entrepreneurial firms in Africa: A quantile regression approach. Small Business Economics, 34(1), 3151.
Greene, W. H. (2012). Econometric Analysis (7th ed.). Pearson Education.
Haltiwanger, J., Jarmin, R. S., Kulick, R., and Miranda, J. (2016). High Growth Young Firms: Contribution to Job, Output, and Productivity Growth. In J. Haltiwanger, E. Hurst, J. Miranda, and A. Schoar, eds., Measuring Entrepreneurial Businesses: Current Knowledge and Challenges (pp. 1162). Chicago: University of Chicago Press.
Hart, P. E., and Prais, S. J. (1956). The analysis of business concentration: A statistical approach. Journal of the Royal Statistical Society, 119(2), 150191.
Heckman, J. (1979). Sample selection bias as a specification error. Econometrica, 47(1), 153162.
Henrekson, M., and Johansson, D. (2010). Gazelles as job creators: A survey and interpretation of the evidence. Small Business Economics, 35(2), 227–244.
Hymer, S., and Pashigian, P. (1962). Firm size and rate of growth. Journal of Political Economy, 70(6), 556569.
Ipinnaiye, O., Dineen, D., and Lenihan, H. (2017). Drivers of SME performance: A holistic and multivariate approach. Small Business Economics, 48(4), 883911.
Jones, A. M., Koolman, X., and Rice, N. (2006). Health-related non-response in the British Household Panel Survey and European Community Household Panel: Using inverse-probability-weighted estimators in non-linear models. Journal of the Royal Statistical Society. Series A (Statistics in Society), 169(3), 543569.
Kerr, S. P., Kerr, W. R., and Xu, T. (2017). Personality Traits of Entrepreneurs: A Review of Recent Literature. Cambridge: National Bureau of Economic Research.
Kozubowski, T., and Nadarajah, S. (2010). Multitude of Laplace distributions. Statistical Papers, 51(1), 127148.
Lawless, M. (2014). Age or size? Contributions to job creation. Small Business Economics, 42(4), 815830.
Lee, H., Smith, K. G., and Grimm, C. M. (2003). The effect of new product radicality and scope on the extent and speed of innovation diffusion. Journal of Management, 29(5), 753768.
Little, I. M. (1962). Higgledy piggledy growth. Bulletin of the Oxford University Institute of Statistics, 24(4), 387412.
Liu, J.-T., Tsou, M.-W., and Hammitt, J. K. (1999). Do small plants grow faster? Evidence from the Taiwan electronics industry. Economics Letters, 65(1), 121129.
López-García, P., and Puente, S. (2012). What makes a high-growth firm? A dynamic probit analysis using Spanish firm-level data. Small Business Economics, 39(4), 10291041.
Lotti, F., Santarelli, E., and Vivarelli, M. (2003). Does Gibrat's law hold among young, small firms? Journal of Evolutianory Economics, 13(3), 213235.
Mathew, N. (2017). Drivers of firm growth: Micro-evidence from Indian manufacturing. Journal of Evolutionary Economics, 27(3), 585611.
Navaretti, G. B., Castellani, D., and Pieri, F. (2014). Age and firm growth: Evidence from three European countries. Small Business Economics, 43(4), 823837.
Park, Y., Shin, J., and Kim, T. (2010). Firm size, age, industrial networking, and growth: A case of the Korean manufacturing industry. Small Business Economics, 35(2), 153168.
Reichstein, T., and Dahl, M. S. (2004). Are firm growth rates random? Analysing patterns and dependencies. International Review of Applied Economics, 18(2), 225246.
Reichstein, T., and Jensen, M. (2005). Firm size and firm growth rate distributions — The case of Denmark. Industrial and Corporate Change, 14(6), 11451166.
Reichstein, T., Dahl, M. S., Ebersberger, B., and Jensen, M. B. (2010). The devil dwells in the tails: A quantile regression approach to firm growth. Journal of Evolutionary Economics, 20(2), 219231.
Rivard, P. (2020). High-Growth Firm Characteristics in Canada. Ottawa: Innovation, Science and Economic Development Canada.
Royston, P. (1991). sg 3.6: A response to sg 3.3: Comment on tests of normality. Stata Technical Bulletin 4: 89, 1, 112114.
Santarelli, E., Klomp, L., and Thurik, A. R. (2006). Gibrat's Law: An Overview of the Empirical Literature. In Entrepreneurship, Growth, and Innovation (pp. 4173). Springer.
Schoonjans, B., Van Cauwenberge, P., and Bauwhede, H. V. (2013). Formal business networking and SME growth. Small Business Economics, 41(1), 169181.
Schreyer, P. (2000). High-Growth Firms and Employment. Paris: OECD Science, Technology and Industry Working Papers.
Seaman, S. R., and White, I. R. (2011). Review of inverse probability weighting for dealing with missing data. Statistical Methods in Medical Research, 22(3), 278295.
Serrasqueiro, Z., Nunes, P. M., Leitão, J., and Armada, M. (2010). Are there non-linearities between SME growth and their determinants? A quantile approach. Industrial and Corporate Change, 19(4), 10711108.
Simon, H. A., and Bonini, C. P. (1958). The size distribution of business firms. American Economic Review, 48(4), 607617.
Stanley, M. R., Amaral, L., Buldyrev, S. V., Havlin, S., Leschhorn, H., Maass, P., Salinger, M. A., and Stanley, H. E. (1996). Scaling behavior in the growth of companies. Nature, 379(29), 804806.
StataCorp. (2013). Stata 13 Base Reference Manual. College Station, Texas: Stata Press.
StataCorp. (2015). Stata 14 Base Reference Manual. College Station, Texas: Stata Press.
Stucki, T. (2013). Success of start-up firms: The role of financial constraints. Industrial and Corporate Change, 23(1), 2564.
Sutton, J. (1997). Gibrat's legacy. Journal of Economic Literature, 35(1), 4059.
Wagner, J. (1992). Firm size, firm growth, and persistence of chance: Testing Gibrat's law with establishment data from Lower Saxony, 1978–1989. Small Business Economics, 4(2), 125131.
Wooldridge, J. M. (2002). Inverse probability weighted M-estimators for sample selection, attrition, and stratification. Portuguese Economic Journal, 1(2), 117139.
Wooldridge, J. M. (2010). Econometric Analysis of Cross section and Panel Data. The MIT Press.